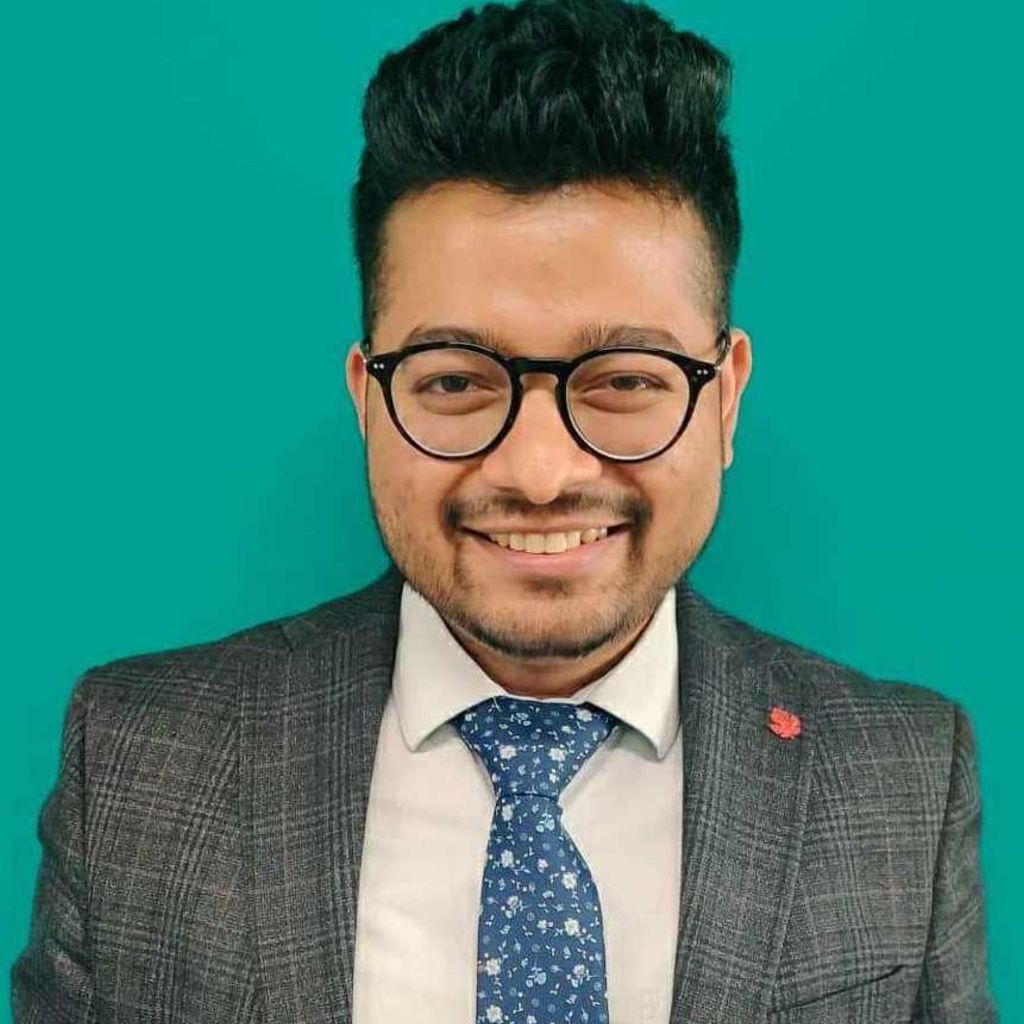
Speaker
Jyotikrishna Dass
Abstract
With the rapid increase in data collected from various edge devices across distributed networks, there is a pressing need for innovative solutions to harness intelligence at the network edge. Traditional cloud-based centralized learning methods won’t suffice. Instead, federated learning, an emerging approach, keeps data local at its source, avoiding the need for centralization on a cloud server. This method pushes model updates to the edge and aggregates local updates to train a global model on a shared parameter server. However, federated learning presents challenges, including poor model convergence compared to centralized learning and device lag due to heterogeneity and network unreliability. To empower distributed edge intelligence efficiently, optimizing machine learning models to utilize decentralized data, adapting to diverse device capabilities, and complying with network constraints are crucial. In this talk, I will delve deeper into these insights and share my research on bridging the gap between centralized and federated learning. My strategy encompasses three key areas: (i) enhancing processor utilization through relaxed synchronization and tackling memory-efficient problems in distributed networks, (ii) developing parallel algorithms that accelerate model learning via data summaries and facilitate linear scaling in decentralized machine learning, and (iii) co-designing energy-efficient systems that make AI accessible at the edge, promoting green AI. To wrap up my talk, I will share some intriguing directions for future research.
Bio
Dr. Jyotikrishna Dass is a Research Scientist at Rice University, where he coordinates the activities at the Center for Transforming Data to Knowledge. He obtained his B.Tech from the Indian Institute of Technology at Guwahati and earned his Ph.D. in Computer Engineering from Texas A&M University (TAMU) in 2021. Subsequently, he held the Postdoctoral Research Associate position at Rice University, where he wrote successful grant proposals for NSF Core Programs ($1.2 million), META Network for AI ($50K), and Rice University Creative Ventures Fund ($10K). His research lies at the intersection of machine learning, parallel and distributed computing, and computer systems, with a particular emphasis on developing distributed algorithms for machine learning and energy-efficient systems. His work has been published in top-tier machine learning and systems venues, including ICML, ICDCS, IPDPS, TPDS, HPCA, Micro, and TC. Dr. Dass represented TAMU at the Annual Computing Conference’19, where his research was recognized with the Best Ph.D. Dissertation Poster Award amongst fourteen SEC universities. Dr. Dass has been honored with the College of Engineering Graduate Teaching Fellow’20 and received the Computer Science & Engineering Teaching Assistant Excellence Award’18 at TAMU.