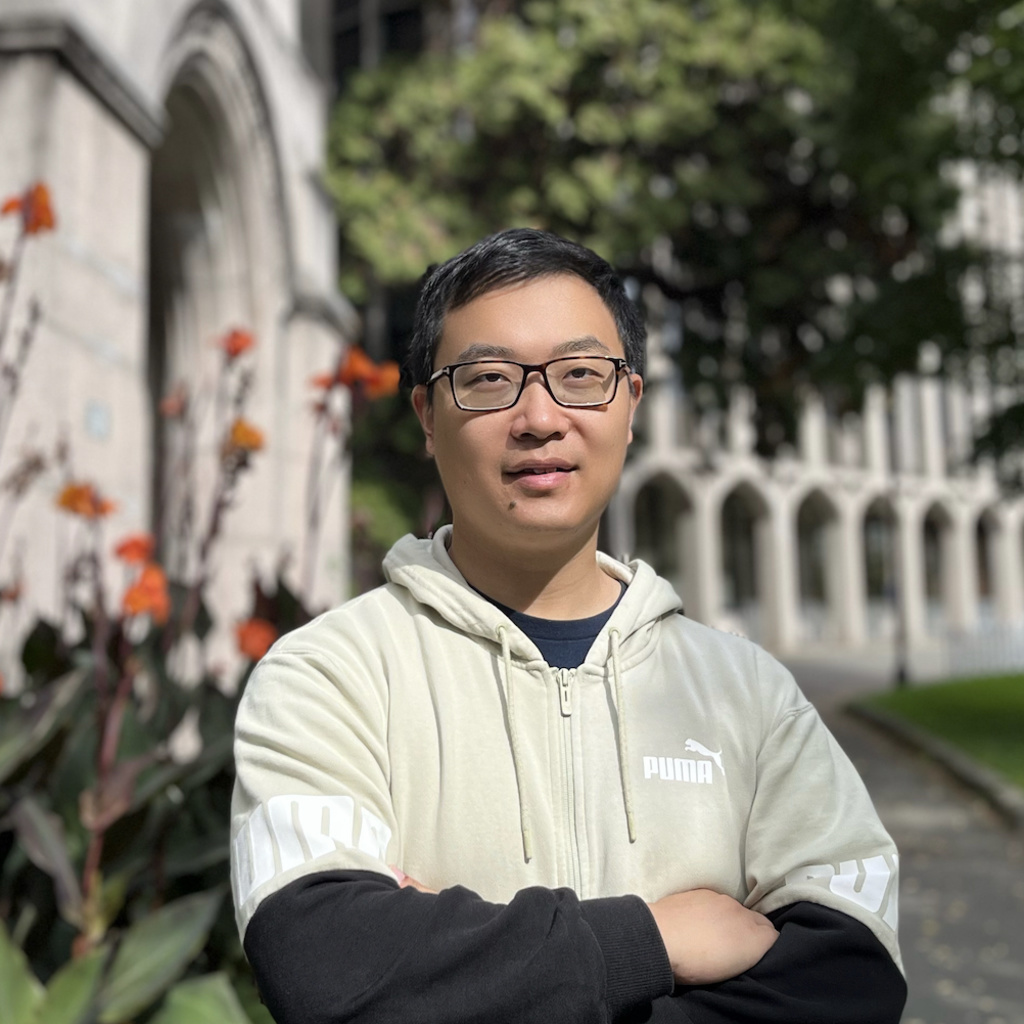
Speaker
Lingxiao Wang
Abstract
The past decade has witnessed rapid growth and tremendous success in machine learning (ML) across various applications, particularly in those involving large ML models trained on vast amounts of data. However, these advancements also raise significant privacy, security, fairness, and copyright concerns.
In this talk, I will talk about my efforts toward addressing these concerns through two important problems: federated optimization and over-parameterized learning. Federated learning (FL) is a framework that prioritizes privacy by allowing multiple clients to keep their sensitive data locally and communicate with a server to train a model collaboratively. While promising, federated optimization methods often suffer from substantial communication costs and do not offer protection against privacy attacks, such as data reconstruction attacks. In the first part of my talk, I will introduce a communication-efficient algorithm for FL and study its optimality. Furthermore, I will discuss strategies for achieving strong privacy protections with our proposed algorithm. In the second part of my talk, I will present our work on training the first over-parameterized linear models with strong privacy and utility guarantees. This demonstrates the potential of our method for training effective large models while mitigating privacy concerns. To conclude, I will discuss future directions toward developing more responsible ML methods and systems.
Bio
Dr. Lingxiao Wang is currently a Research Assistant Professor at Toyota Technological Institute at Chicago (TTIC). He obtained his PhD from the Department of Computer Science at the University of California Los Angeles (UCLA), where he was advised by Quanquan Gu. Previously, he obtained his MS degree in Statistics at the University of Washington. Lingxiao's research is broadly in machine learning, including privacy-preserving machine learning, collaborative learning (federated and distributed learning), low-rank matrix learning, and high-dimensional statistics. He is a recipient of Rising Stars in Data Science from the University of Chicago. His research has also been recognized with the best paper award at ICML 2023 FL workshop.