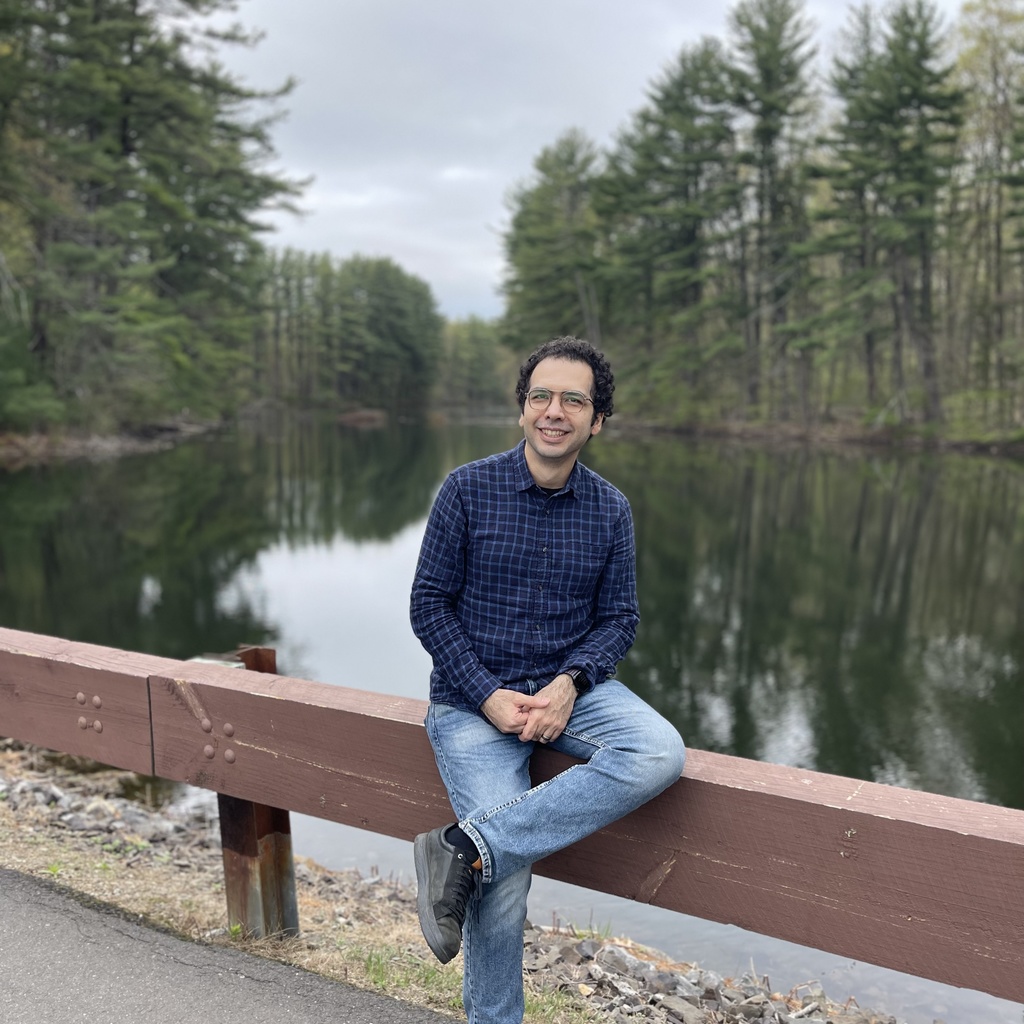
Speaker
Amir Ghasemian
Abstract
Networks have attracted wide interest in the scientific community as a powerful tool for studying complex systems. In this talk, I answer important questions about the limitations of model selection, link prediction, and community detection in complex networks. Although many community detection algorithms exist, the No Free Lunch theorem implies that no algorithm can be optimal across all inputs. For example, little is known in practice about how different community detection algorithms over- or under-fit to real networks, or it has remained unknown whether a single best link predictor exists, how link predictability varies across methods and networks from different domains, and how close to optimality current methods are.
I present a broad investigation of over- and under-fitting across 16 state-of-the-art community detection algorithms applied to a benchmark corpus of 572 structurally diverse real-world networks. I also answer questions about optimal link prediction by systematically evaluating 203 individual link predictor algorithms applied to this corpus. I first show that individual algorithms exhibit a broad diversity of prediction errors, and then exploiting this diversity using network-based metalearning, I construct a series of “stacked” models that combine predictors into a single algorithm that achieves optimal or nearly optimal levels of accuracy. Additionally, I show how this approach can be extended efficiently to temporal networks.
Bio
Amir Ghasemian is a research scientist in the computational social science lab led by Duncan Watts at the University of Pennsylvania. Previously, he was a post-doctoral research associate at Yale University's Human Nature Lab, led by Nicholas Christakis. He received his PhD from the University of Colorado Boulder, working with Aaron Clauset. His research interests lie in network science, statistical inference, causal inference, statistical physics, and machine learning. Dr. Ghasemian is the recipient of the NSF/CRA/CCC Computing Innovation Award (CIFellow 2020), and his research has been published in top physics, computer science, and multidisciplinary scientific journals such as Physical Review X, TKDE, and PNAS.