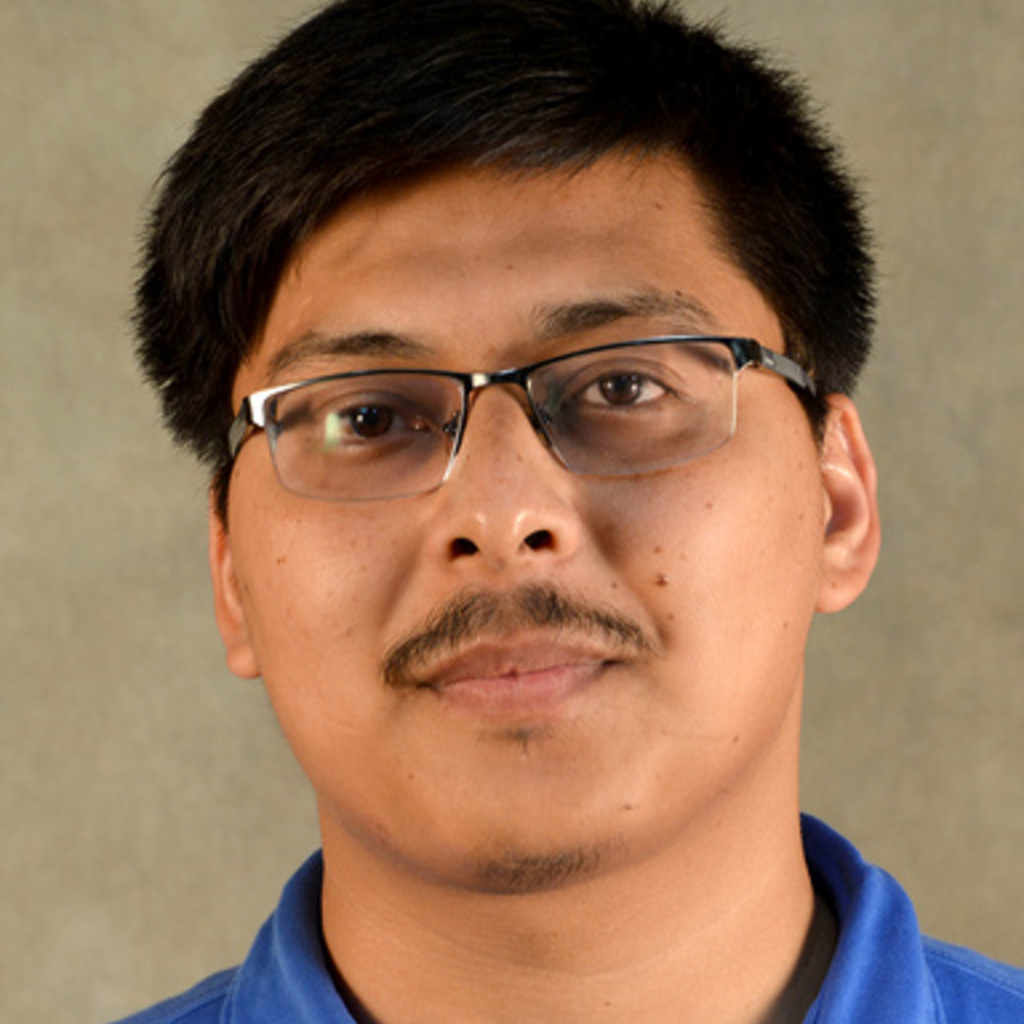
PhD Candidate: Ingroj Shrestha
Abstract
Advancements in language models have significantly improved the generation of coherent text. These models, alongside other neural network-based systems, have found success in various downstream applications within Natural Language Processing (NLP), including text classification and chatbot systems, thereby being integrated into various applications. However, a notable challenge arises as these models are susceptible to inheriting and perpetuating biases from their training data, particularly against certain demographics, such as gender and race, within specific contexts like professions and behavioral concepts. This underscores the importance of detecting bias within these systems and implementing measures to mitigate its impact. While there is a sizeable body of research on bias, detection, and mitigation, there are still important problems to be addressed. We extend this body of research in the following directions: (1) human character traits and (2) leadership rating of public figures (3) we also introduce novel methodologies for detecting and mitigating bias, highlighting gaps in existing approaches. Our proposed approach introduces novel methods for detecting bias in upstream text generation systems, namely, Masked Language Models (MLMs) and Autoregressive Language Models (ALMs). In addition, we present strategies to mitigate bias in the upstream text generation systems and in downstream applications, specifically text classification systems.
Advisor: Padmini Srinivasan