Department of Computer Science faculty and graduate students attended the Society for Industrial and Applied Mathematics International Conference on Data Mining (SDM25) earlier this month to present their research. Professor Bijaya Adhikari co-authored three papers accepted to the conference and was joined by two of his collaborators and advisees, Ph.D. candidates Akash Choudhuri and Hieu Vu. He was also one of the keynote speakers at the conference's 3rd Data Science for Smart Manufacturing and Healthcare Workshop, in which he presented "Data Mining Electronic Health Records to Combat Healthcare-Associated Infections". On the final day of the conference, Professor Muchao Ye hosted a minitutorial entitled "Exploitation and Mitigation: Understanding Large-Scale Machine Learning Robustness under Paradigm Shift".
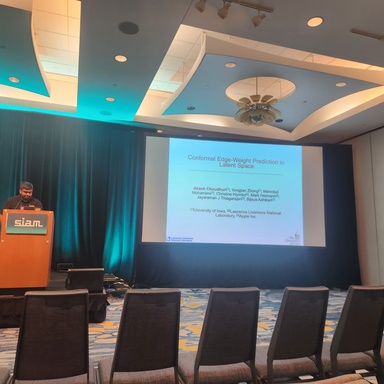
Choudhuri collaborated with Adhikari and was the first author of the papers "Domain Knowledge Augmented Contrastive Learning on Dynamic Hypergraphs for Improved Health Risk Prediction" and "Conformal Edge-Weight Prediction in Latent Space". Vu also co-authored the latter. Adhikari's paper, "Accurately Estimating Unreported Infections using Information Theory", was also accepted to the conference.
Read on for insights into each of the accepted papers, workshops, and the participants' experiences from the conference:
Accepted Papers
- "Domain Knowledge Augmented Contrastive Learning on Dynamic Hypergraphs for Improved Health Risk Prediction" Akash Choudhuri, Hieu Vu, Kishlay Jha, and Bijaya Adhikari
[This research] is motivated by the importance of physical interactions (patient-healthcare worker contact, patient-room contact) and the prescriptions of medications to patients during hospital stays for accurate estimation of patient risk. We model these interactions through hypergraphs, where each hyperedge models the higher order interactions amongst a group of patients (healthcare-worker that interacts with them/ room they were transferred to/ medication they were prescribed). Additionally, we leverage domain information in the form of healthcare-worker specialities and medication hierarchies and use them to propose contrastive augmentations that lead to more domain-aligned and robust patient embeddings. This work is motivated through the mode of the spread of infectious diseases where pairwise relationships are not enough to model the spread. Additionally, the joint training of higher-order patient interactions and temporal aggregation of patient risks allows our framework to be applied for other tasks like MICU transfer prediction, the results of which are provided in the paper. "
-Akash Choudhuri
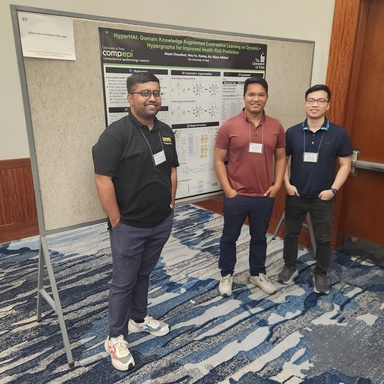
"From my perspective, the main challenge was not technical difficulties but understanding the limitations of existing literature. We found that no prior studies had combined high-order patient interactions, domain knowledge (such as medications and physician expertise), and the temporal nature of clinical data. Our work creatively integrates these features and successfully improved the quality of the predictions for two important tasks: CDI Incidence Prediction and MICU Transfer Prediction. We recognize that accurate health risk prediction is crucial for making in-formed clinical decisions and assessing the appropriate allocation of medical resources. Through our research, we hope to contribute to the improvement of healthcare systems by leveraging data-driven and machine learning techniques."
-Hieu Vu
- "Conformal Edge-Weight Prediction in Latent Space" Akash Choudhuri, Yongjian Zhong, Mehrdad Moharrami, Christine Klymko, Mark Heimann, Jaya Thiagarajan and Bijaya Adhikari
"[This paper] uses a statistical technique known as conformal inference to the edge-weight prediction task in graphs. This work — which me and Yongjian started working on during our internship at LLNL (Lawrence Livermore National Laboratory)— constructs a non-conformity score (a heuristic notion of predictive error) on the latent node space and transforms this notion of uncertainty to the edge space with a band estimator operator. This research is extremely significant as edge-weight prediction tasks are particularly relevant in datasets using biological datasets and uncertainty quantification in this field reduces the number of trials required for drug discovery research."
-Akash Choudhuri
- "Accurately Estimating Unreported Infections using Information Theory" Jiaming Ciu, Bijaya Adhikari, Arash Haddadan, A S M Ahsan-UI Haque, Jilles Vreeken, Anil Vullikanti and B. Aditya Prakash
"This paper focuses on inferring latent/missing/asymptomatic infection counts from observed reported infection counts. It is crucial to infer the total number of infections during an epidemic outbreak for appropriate resource allocation and to design effective intervention strategies. We leveraged an information-theoretic framework built on the standard epidemiological model to create our approach. The proposed approach was highly accurate in estimating total infections in real data."
-Bijaya Adhikari
Q&A with Iowa's SDM25 participants
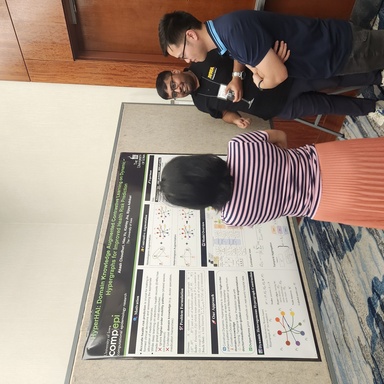
What was your favorite moment (or a memorable experience) from the conference? Were there any projects or presentations that stuck out to you?
Adhikari: My favorite part about attending this conference was that the University of Iowa was well represented. Four members (two faculty members and two students) attended.
Choudhuri: I enjoyed interacting with fellow PhD students who were in different schools all over the world during poster sessions and breaks. I see them as my potential future collaborators. SDM provided me with the opportunity to have these interactions and brought several renowned researchers and faculty working in the field of data mining. Particularly, a related work was presented by Zohair, a PhD student at Northeastern University that really caught my attention. I had a great time talking to Zohair after his presentation.
Vu: My favorite moment was the "Doctoral Forum Best Poster Award" session. It was inspiring to see rising stars in the data mining research community, which motivated me to aim higher. Hopefully, I can be among them next year.
One project that I was impressed with was a poster with an interactive demo on a tablet, which is a very creative way to showcase the study put into real-life applications.
Ye: My favorite moment was attending the conference with the group of Dr. Bijaya Adhikari. It’s my first time attending a conference since I've worked here and it’s a joyful moment that I can go to a conference and represent our department with other brilliant researchers like Bijaya together.
What workshops or events did you participate in?
Adhikari: I gave a keynote address at the 3rd Data Science for Smart Manufacturing and Healthcare Workshop. My talk focused on Data Mining Electronic Health Records to Combat Healthcare-Associated Infections.
Choudhuri: Although I spent most of my time listening to paper presentations, I attended a minitutorial titled ‘Integrating Textual and Graph Data: Advancing Knowledge Discovery with Semantic and Structural Insights’ that was presented by Jiawei Han and his group. Jiawei Han is a pioneer in my field, and [it was] an amazing experience to be able to probe on his research ideas and the perspective of his group towards the future directions of data mining in healthcare research.
Vu: I attended several workshops and tutorials, including "AI for Time Series Analysis: Theory, Algorithms, and Applications" and "Unifying Spectral and Spatial Graph Neural Networks." Both were very engaging and helpful for my research. If I have accepted papers at the conference next year, I will definitely join these sessions again.
Ye: The main event I participated in was giving a tutorial titled Exploitation and Mitigation: Understanding Large-Scale Machine Learning Robustness under Paradigm Shift, related to my research and lectures on adversarial robustness of AI models. This is a tutorial co-organized with Dr. Xi Li from UAB and Dr. Ruixiang Tang from Rutgers. We are looking forward to doing it again. I was attending SDM25 for giving a tutorial there.
What advice would you give to someone planning to submit to or attend SDM in 2026?
Adhikari: SDM is relatively smaller than other Data Mining/AI/Machine Learning conferences. Thus, it provides an excellent opportunity for in-depth networking with members of the data mining community. I recommend students prepare to take advantage of the conference's smaller size.
Choudhuri: For someone planning to submit to SDM 2026, I would say that this conference is a top conference, both in the quality of papers you will share space with if your work gets accepted, and the people you will get to meet. I mean, I was so pumped to find Brian Perozzi from Google presenting a poster in the same room as me! Additionally, SDM is extremely student friendly. I was extremely grateful to be awarded a travel grant funded by the NSF that covered the registration and accommodation fees for the conference. For anyone planning to attend SDM, I would say that this is a conference where some of the most famous researchers in data mining attend the conference and due to a very small number of papers that get accepted, these researchers truly provide you with more intimate and constructive feedback. It is a great place to build your network and learn new things!
Vu: I think in recent years, applied machine learning studies had a greater chance to get accepted to the SDM conference, which is good starting place for graduate students to submit their research without too much in-depth theoretical results. It's also a great place to expand your network in research and industry communities, as this year, many senior researchers from big universities and companies came and shared their great works, as well as their vision on the future of AI.
Be confident in your research ideas; it can turn into great research works. Submit [to SDM26] and let the community evaluate your findings.
Ye: SDM is a great conference connecting with people in the field of data mining. My advice for attending SDM 2026 would be enjoying connecting with people in the conference and keeping learning from other researchers.