Mehrdad Moharrami, Ph.D.
Moharrami is an Assistant Professor in Computer Science at the University of Iowa. Previously, he was a TRIPODS Postdoctoral Research Fellow at UIUC, working with Prof. R. Srikant. He earned his BSc in Mathematics and Electrical Engineering from the Sharif University of Technology, Iran, and holds MSc degrees in Electrical Engineering and Mathematics from the University of Michigan. In Winter 2020, he received his PhD in Electrical Engineering, earning the prestigious Rackham Predoctoral Fellowship for his exceptional dissertation.
Research Interests
His research encompasses reinforcement learning, MDPs, and computationally efficient algorithms for learning in societal systems modeled as random graphs. His work has been published in top Applied math Journals and Computer Science Conferences.
- Algorithmic Foundations
- Social Computing and Public Policy
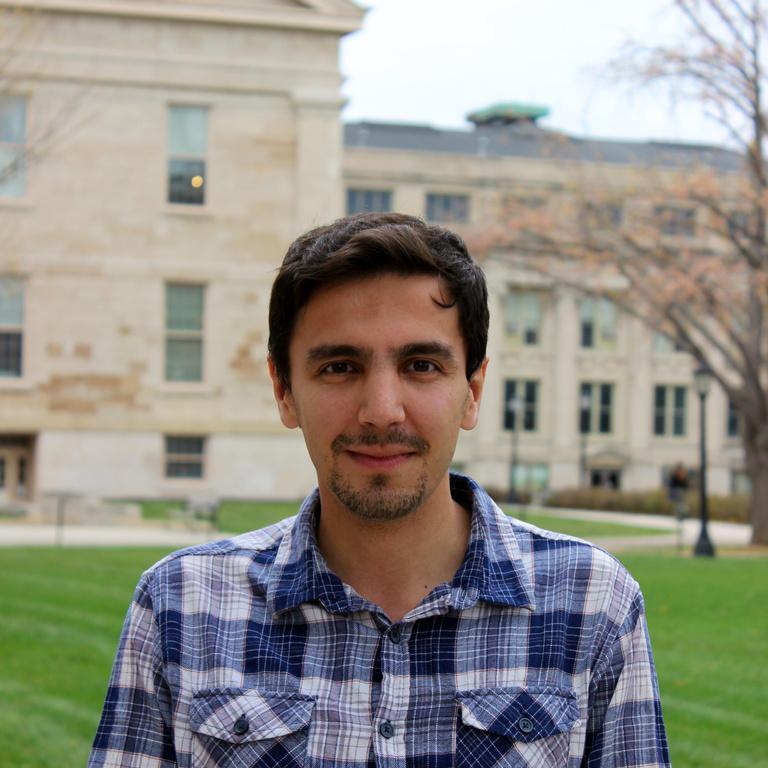